Wouter A. H. Spekkink
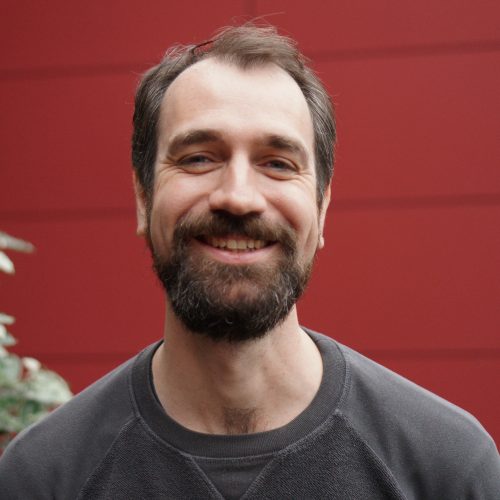
T17-15 (Mandeville)
Burgemeester Oudlaan 50
3062 PA, Rotterdam
I am currently an Assistant Professor at the Department of Public Administration and Sociology of the Erasmus University Rotterdam. I am also part of the Erasmus Governance Design Studio. I do research on various topics related to the governance of sustainability. My teaching focuses mostly on governance in the public sector.
news
Aug 2, 2023 | Finally added a new blog post after another long break. |
---|---|
Jul 31, 2023 | I switched to a new website template. This is partly work in progress. |
latest posts
Oct 23, 2023 | Retrieval augmentation with literature |
---|---|
Aug 3, 2023 | Simple universal bookmarking |
Aug 2, 2023 | Using LLMs with retrieval augmentation through LangChain |